Can transformers be used as privacy preserving techniques? A case of study: Mobile Sensors
Introduction
Mobile sensors, like smartphones, smartwatches, and other wearables, are being used increasingly often in our daily lives. Each person's location, physical activity, heart rate, and other data are collected by these devices. However, since mobile sensors are used more often, there is a greater need for privacy-preserving methods to safeguard people's private information. We will examine how artificial intelligence (AI) may be utilised to protect privacy in mobile sensor data in this blog post.
The Importance of Privacy-Preserving Techniques in Mobile Sensors
As mobile sensors capture more and more data on individuals, the need for privacy-preserving approaches becomes more pressing. Sensitive information, such as location data and personal health information, is frequently collected via mobile sensors. If this information falls into the hands of the wrong people, it might be used for unscrupulous objectives such as identity theft or targeted advertising. Individuals may also be concerned about their data being used to make choices about them, such as in insurance or employment. It is critical to guarantee that sensitive data is safeguarded and not exploited.
Furthermore, as IoT has grown in popularity and the number of connected devices has grown, the volume of data created has skyrocketed. This data is frequently utilised for a variety of purposes, including customization, analytics, and decision-making. However, the information gathered by these devices frequently contains sensitive information about the individuals and their surroundings. As a result, developing privacy-preserving solutions to ensure that this data is not exploited is critical.
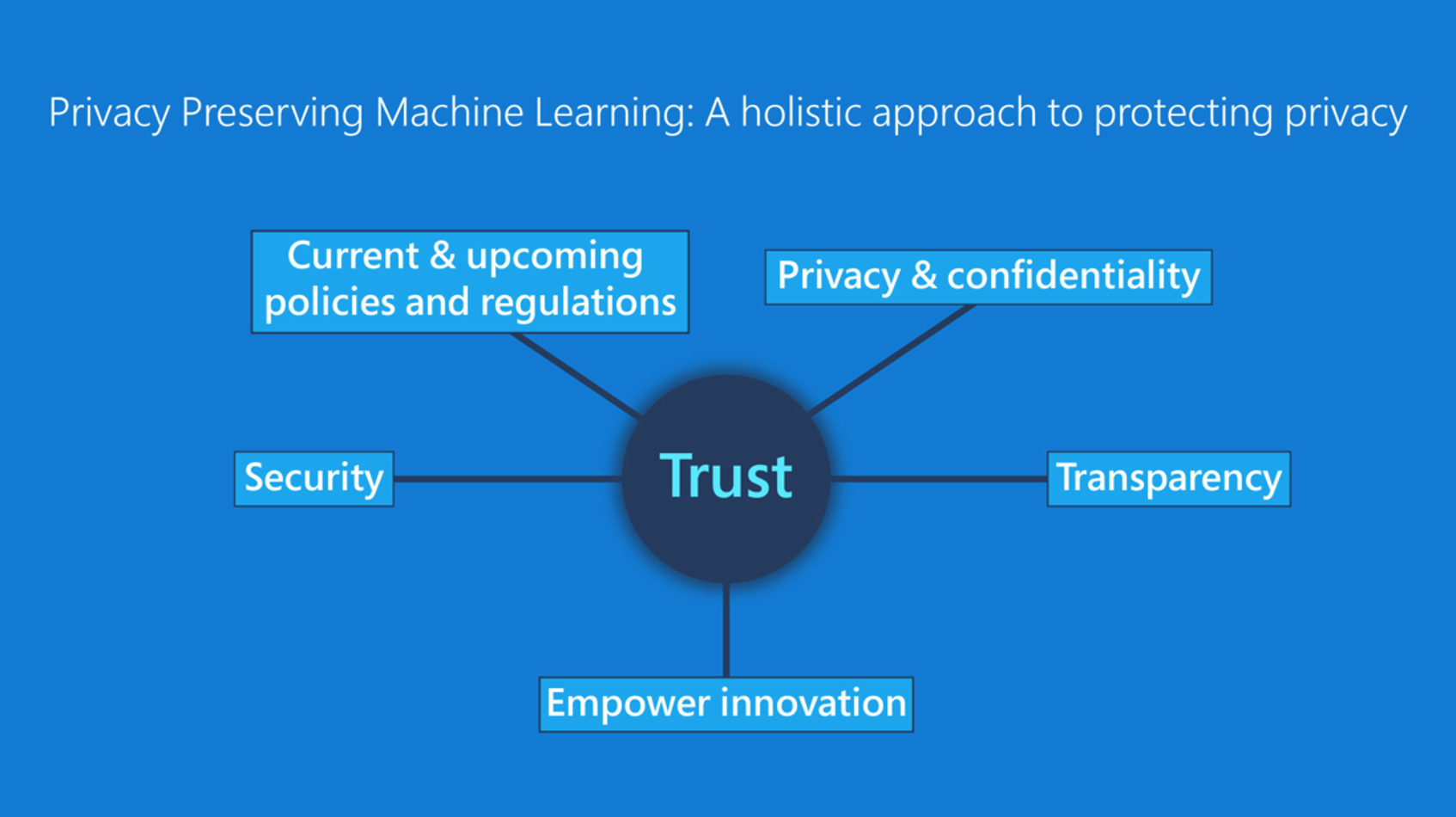
Using Artificial Intelligence for Privacy-Preserving in Mobile Sensors
Using AI to protect privacy in mobile sensor data is one way. AI-based models may be used to examine sensor data while remaining anonymous. A transformer-based model, for example, can be used to detect abnormalities in sensor data without exposing the data. Furthermore, AI-based models may be employed in mobile sensor data for activity detection, such as detecting human behaviours, without exposing the data. This is one of the most effective ways to protect one's privacy.
Conclusion
The significance of privacy-preserving approaches in mobile sensors has been discussed in this blog post. In addition, how AI may be utilised to protect privacy in mobile sensor data. As mobile sensors collect more data on individuals, it is critical to create privacy-preserving strategies to secure individuals' sensitive data. AI-based models offer a promising way to reaching this aim, but further study is required to fully realise their potential.
Recommended resources:
[1] EU 2016/679 (General Data Protection Regulation), https:// gdpr-info.eu/.
[2] P. Delgado-Santos, G. Stragapede, et al. "A Survey of Privacy Vulnerabilities of Mobile Device Sensors." arXiv preprint arXiv:2106.10154 (2021), https://arxiv.org/pdf/2106.10154.pdf
[3] A. Henriksen et al. "Using Fitness Trackers and Smartwatches to Measure Physical Activity in Research: Analysis of Consumer Wrist-worn Wearables." Journal of medical Internet Research
[4] E. Murnane et al. “Mobile and Sensor Technology as a Tool for Health Measurement, Management, and Research with Aging Populations”. National Academies of Sciences, Engineering, and Medicine (2020)
[5] C. Gupta, et al. "A Systematic Review on Machine Learning and Deep Learning Models for Electronic Information Security in Mobile Networks." Sensors 22.5 (2022): 2017.
This blogpost was written by Paula Delgado-Santos. She received the M.Sc. degree in Telecommunications Engineering from Universidad Autonoma de Madrid, Spain, in 2020. At the same time, she was working in a scholarship of IBM. In 2019/2020 she was working at a Swiss University, HEIG-VD, as a Data Scientist. In 2020 she started her PhD with a Marie Curie Fellowship within the PriMa (Privacy Matters) EU project at University of Kent, United Kingdom. Her research interests include signal and image processing, pattern recognition, machine learning, biometrics and data protection.
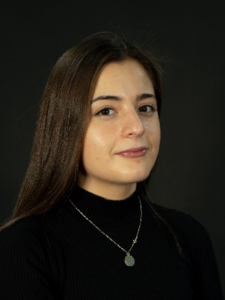